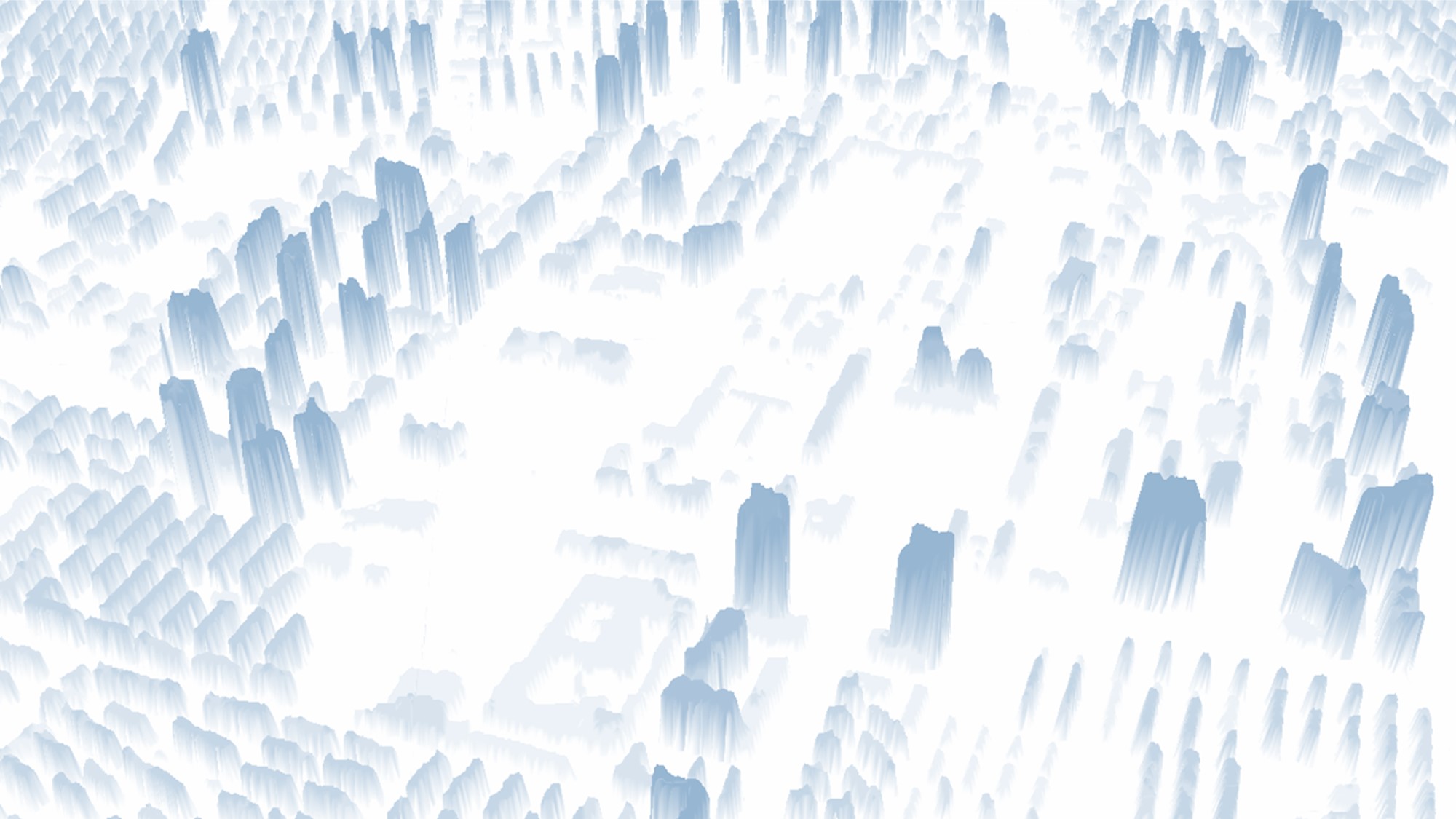
城市建筑轮廓和高度等信息是城市研究和规划的基础数据之一,但实际工作中很难大范围获取。
基于高精度卫星影像数据和深度学习的建筑识别技术的大规模应用,为大范围、快速、较准确的识别分析城市三维建筑提供了新的思路。
3D buildings are crucial for urban studies and planning. However, it is usually not publicly accessible in China.
The development of deep learning provides a new approach for extracting 3D buildings quickly, accurately (to-some-extent), and in a large scale.
技术框架 Methodology
我们首先将部分矢量建筑与多年份卫星图叠合,人工筛选出其中吻合准确的图片。将它们与开源数据集混合,作为训练集。然后基于deeplabV3+微调并训练了建筑轮廓提取模型、建筑层高预测模型。最后将训练的模型应用到上海高精度卫星影像,提取获得三维建筑。
Firstly, we superposed vector buildings with satellite images and screened out the accurate images manually as training sets. Then building footprint extraction model and the height prediction model were trained. Finally, the modeled were combined to obtain three-dimensional buildings.
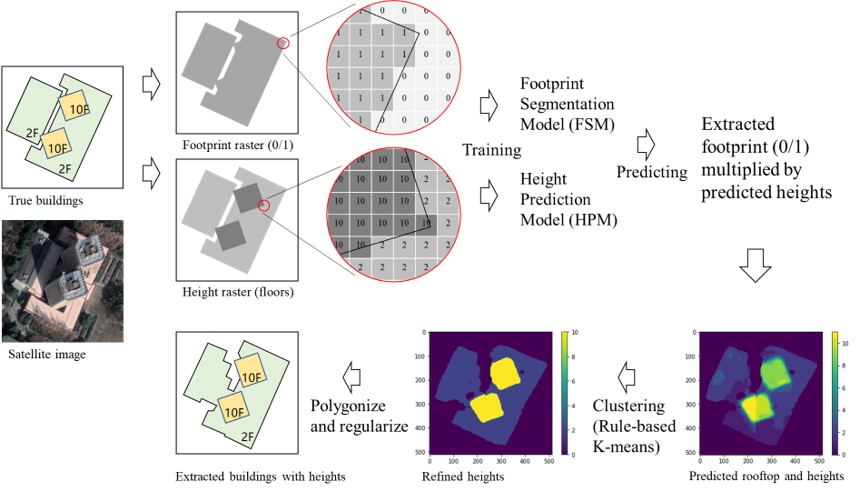
识别效果 Model accuracy
训练的建筑轮廓提取模型,在测试集上的像素预测精度(Precision)高达92%以上,IoU高达0.74,建筑基底面积误差比率不超过5%,能够较好的提取建筑轮廓。
训练获得的建筑层高预测模型,在测试集上的像素层高预测误差控制在0.87层以下,建筑面积误差比率不超过10%。提取结果能够较好的反映城市建筑面积空间分布和基本的三维形态。
The building extraction model has a pixel-wised Precision ≥ 92%, an IoU ≥ 0.7, and a MAPE < 5% on the test set.
The height prediction model obtained has a pixel-wised floor height MAE ≤ 0.87 floor and a MAPE of building area< 10% on the test set, indicating usabilities of both the models.
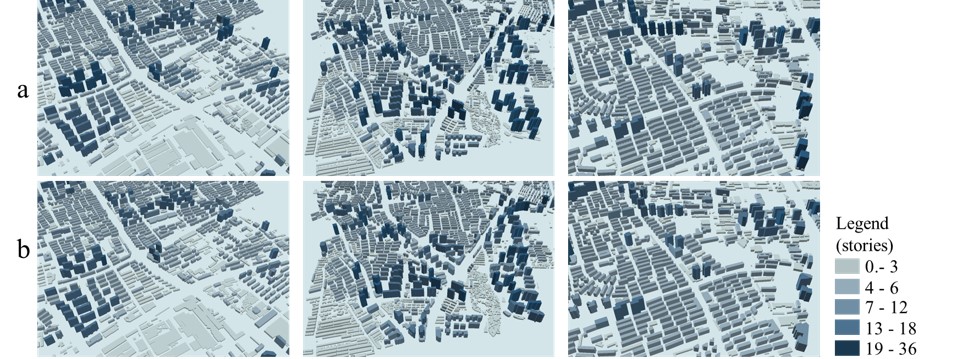
用于光伏潜力估计 estimating of urban-scale photovoltaic potential
提取的三维建筑可以用于城市光伏潜力的估计。下图是采用提取的三维建筑模拟分析的大尺度建筑表面光伏潜力分布图。
定量比较发现,两者差异很小,说明至少在这一任务上提取的矢量三维建筑能够较好的代替测绘的建筑,应用到各种类似的城市三维建模任务中。
PV potentials in typical areas were estimated and compared using both extracted and realistic 3D buildings.
The estimated PV potentials derived from the actual and predicted building models showed little difference, implying the reliability of the estimated PV distribution.
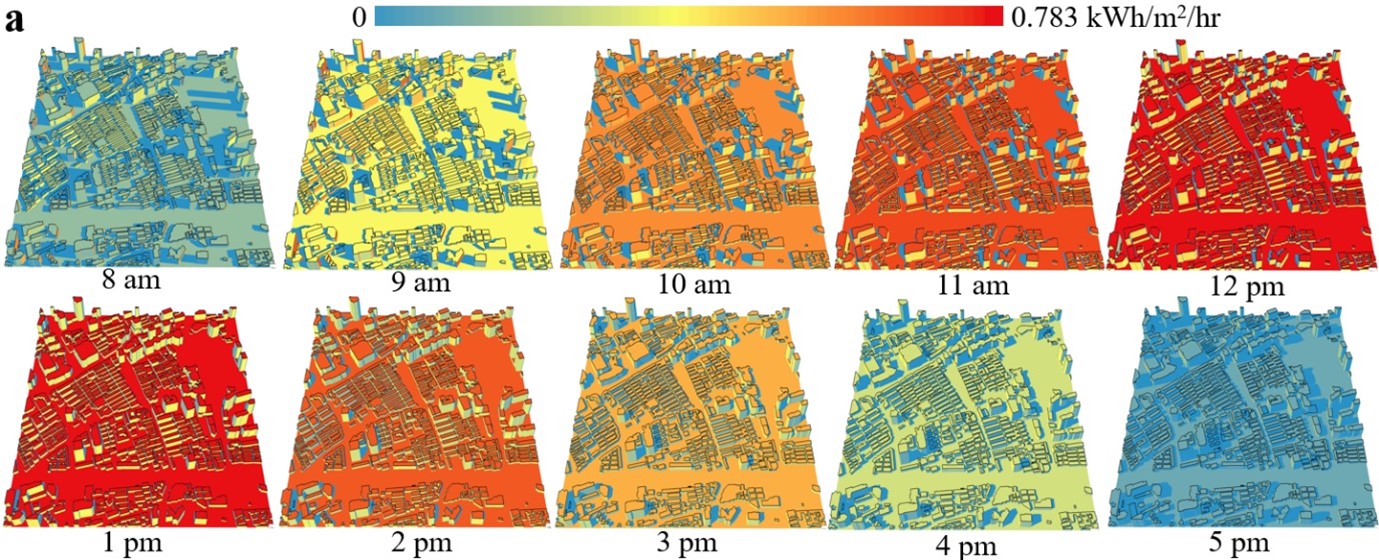
Ref:
晏龙旭,等. 基于深度学习的建筑识别技术在城市体检中的应用[J].上海城市规划, 2022 (01):39-46.
Longxu Yan, et al. Estimation of urban-scale photovoltaic potential: A deep learning-based approach for constructing three-dimensional building models from optical remote sensing imagery. Sustainable Cities and Society (2023): 104515.